Yu (Demi) Qin
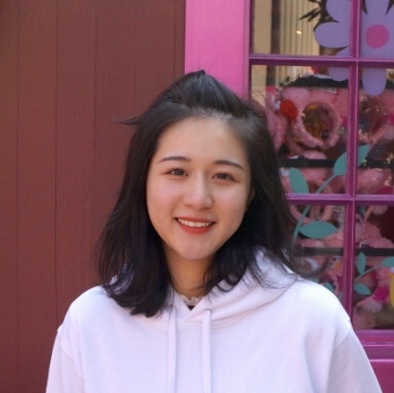
Hello! Welcome to the space of 秦瑜, and you can call me Demi 👋
I’m a recent Ph.D. in Computer Science at Tulane University, specializing in machine learning, topological data analysis, and advanced visualization. My dissertation, titled Metric Learning on Topological Descriptors is advised by Prof. Brian Summa and Prof. Carola Wenk.
My research falls at the intersection of machine learning (ML), visualization (VIS) and topological data analysis (TDA). I aim to push the boundaries of how we understand and interpret complex data efficiently. Using advanced visualization techniques and ML, I enhance large data analysis and explore the shapes and geometries of complex datasets, from scalar fields and images to 3D shapes and graphs. These techniques enable scalable data capture and analysis, potentially improving decisions that affect billions daily.
My work finds applications in diverse areas: enhancing medical imaging for better diagnostics, advancing climate models for precise weather forecasting and climate change analysis, and increasing efficiency in supply chain management.
news
Jan 19, 2025 | 🌟 Our paper “Learning production functions for supply chains with graph neural networks” has been accpeted for an oral presentation at AAAI 2025 (top 5%)! |
---|---|
Nov 04, 2024 | 🎓 I defended my dissertation, “Metric Learning on Topological Descriptors”! Special thanks to my thesis committee and all the wonderful people who have supported me throughout my PhD. |
Aug 08, 2024 | 🎉 We got the Best Paper Award at VIS 2024 (top 1%)! I’m honored to be giving a plenary talk right after the opening ceremony. |
Jul 29, 2024 | 🌟 Our paper “Rapid and Precise Topological Comparison with Merge Tree Neural Networks” has been accpeted by IEEE VIS with an acceptance rate of 22.26%! The paper will be published in the special issue of the IEEE TVCG jounral. Looking forward to seeing everyone in St. Pete Beach. |
Jul 15, 2024 | 🛎 I’m participating in the ICML Topological Deep Learning Challenge 2024! I’m proposing a novel topological lifting approach based on node attributes to convert a graph into a hypergraph. Check out our implementation details here. |